Table Of Content

Drug development and discovery includes preclinical research on cell-based and animal models and clinical trials on humans, and finally move forward to the step of obtaining regulatory approval in order to market the drug. Once a compound that fulfills all of these requirements has been identified, it will begin the process of drug development prior to clinical trials. Next, firstly let us consider some examples of drug-target interaction identification methods. DeepLPI method [17] focused solely on drug SMILES (Simplified Molecular Input Line Entry System) [18] and target sequences. It employed a ResNet-based 1-dimensional convolutional neural network (1D-CNN) to extract sequence features from drug and target. These sequence features were then fused using a bi-directional long short term memory network (biLSTM) and inputed into a FC network to predict drug-target interaction.
Absolute free binding energy calculations
The increasing volume of available data has given a strong impulse to computer-aided drug design, with the latest developments focused on the applications of deep learning (DL) [30,31]. These methods take advantage of the already known concept of artificial neural networks and, due to the augmented performance of calculators, increase their complexity, reaching a much improved performance compared to other ML algorithms [32,33,34]. Other recent studies that benchmarked DL against other machine learning algorithms for properties prediction, using large biomolecular datasets comprising hundreds of thousands of compounds, consistently showed that deep neural networks are the best performing approach [42,43]. In addition to properties prediction or screening, DL has been employed in de novo design. With this machinery, novel structures were proposed that showed potential specific anticancer properties [45] and a predicted activity against dopamine receptor type 2 [46]. Analogous approaches employing the power of DL have been used to develop tools for the design of a molecule that can adapt best to a given 3D protein pocket [47] or that can display a particular desired property [48].
Mass spectrometry analysis
A main problem to be resolved for precision medicine is the development of effective therapies targeted at the central nervous system (CNS). Some of the approaches explored to address this challenge include blood–brain barrier disruption and drug modifications to enhance CNS permeability; unfortunately, neither approach has proven successful. Another approach is to deliver therapeutics locoregionally, directly into the tumor mass and the surrounding tumor-infiltrated brain parenchyma. The most widely used method for direct brain delivery is convection-enhanced delivery (CED), whereby specially designed catheters are introduced into target tissue, and the infusate is delivered slowly over a prolonged period of time. CED enables the delivery of conventional, nano-, bio-, gene, and even cellular therapies [61,62,63,64,65,66,67,68,69,70,71,72,73].
Activity change score
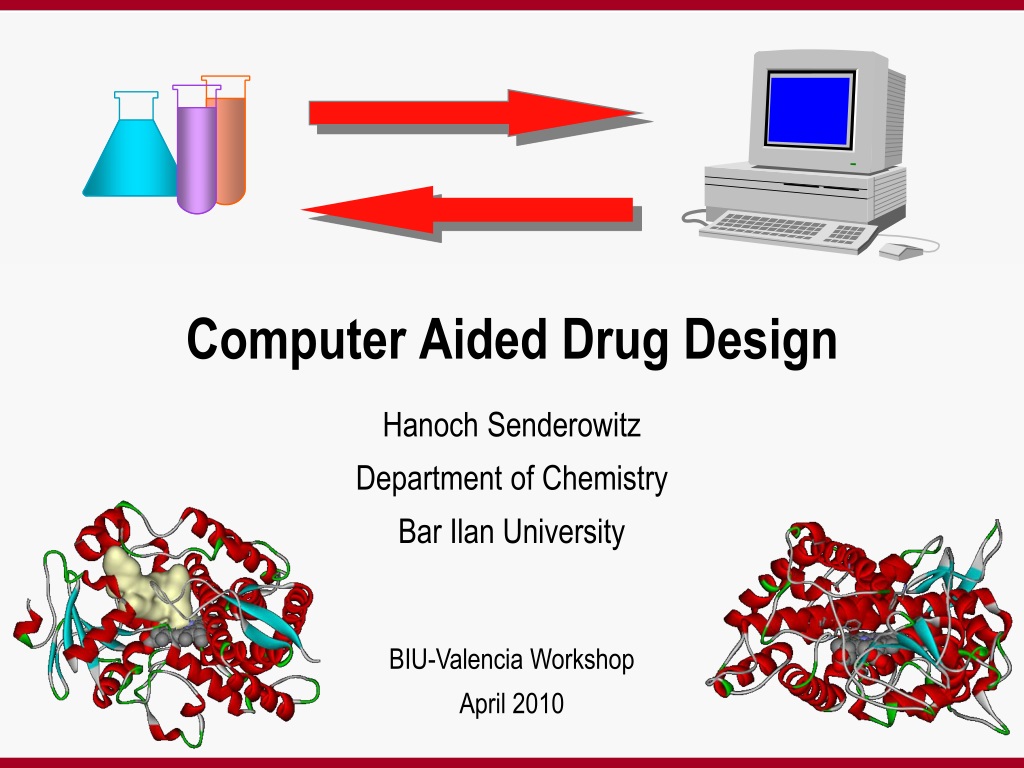
The real test resides with the identiication of such lead real test resides with the identification of such lead bioactive positions on the basic skeleton of such leads. The drug is most commonly an organicsmall molecule that activates or inhibits the function of a biomolecule such as a protein, which in turn results in a therapeutic benefit to the patient. In the most basic sense, drug design involves the design of small molecules that are complementary in shape and charge to the biomolecular target with which they interact and therefore will bind to it. Drug design frequently but not necessarily relies on computer modeling techniques. Finally, drug design that relies on the knowledge of the three-dimensional structure of the biomolecular target is known as structure-based drug design.
Design Based in Making Favorable 3D Interactions¶
Nonrandom screening, also called targeted or focused screening, is a more narrow approach than is random screening. In this case, compounds having a vague resemblance to weakly active compounds uncovered in a random screen, or compounds containing differentfunctional groups than leads, may be tested selectively. By the late 1970s, the National CancerInstitute’s random screen was modified to a nonrandom screen because of budgetary and manpowerrestrictions. Also, the single tumor screen was changed to a variety of tumor screensbecause it was realized that cancer is not just a single disease. “Our work has made the world of proteins accessible for generative AI in drug research,” Schneider says. “The new algorithm has enormous potential.” This is especially true for all medically relevant proteins in the human body that don’t interact with any known chemical compounds.
Part II The Search for the Lead Structure
To obtain a library of candidate ligands, the ligand-binding site of human PPARγ protein (PDB-ID 3G9E41) was utilized as the structural template (Fig. 3a). A total of 300 k molecules were generated, and subsequent filtering was performed based on specific criteria. These filters included an upper molecular weight limit of ≤600 g mol-1, a RAScore threshold of ≥0.5, and a novelty score of ≥0.7.
The first sulphonamide drug Prontosil was discovered by random in vitro screening, when a great number of colorants were screened for antibacterial activity [2,23]. Another approach for drug discovery is by chemical modifications of known drugs or natural products [2,16]. The natural product salicylic acid was acetylated in order to increase the stability and reduce the irritating effect on stomach mucosa. Small chemical modifications lead to improved therapeutic profiles in drugs of different generations.
AlphaFold found thousands of possible psychedelics. Will its predictions help drug discovery? - Nature.com
AlphaFold found thousands of possible psychedelics. Will its predictions help drug discovery?.
Posted: Thu, 18 Jan 2024 08:00:00 GMT [source]
While the negative control compound 222A5 neither disrupted the binding of PTEN and DUSP7 to SPOP, nor decreased the ubiquitination of PTEN and DUSP7 (Supplementary Fig. 2e–h). Due to the inhibition of PTEN and DUSP7 ubiquitination under 230D7 treatment, accumulation of cellular PTEN and DUSP7 proteins was observed in 786-O cells treated with 230D7, causing decreases in phosphorylated AKT and ERK (Fig. 5f, g). Next, we tested the cell proliferation of three ccRCC cell lines (786-O, Caki-2, OS-CR-2) and two non-ccRCC cell lines (4T-1, MDA-MB-231) in the presence of 230D7 (Fig. 5h).
O cells xenograft tumor growth
Her research focuses on the 'use of structural information to design highly potent and selective enzyme inhibitors'. She also serves as chair of the Drug Discovery and Mechanisms of Antimicrobial Resistance Study Section. While exhibiting sufficient structural and scaffold novelty, the generated molecules also possess a topology commonly observed in modulators of the PPAR subfamily, as captured by the three different QSAR models. Specifically, they feature an acidic head group connected to an aromatic core through a linker. Furthermore, this core is linked to another single- or bicyclic aromatic ring system.
Compounds 1–3 were characterized in a hybrid reporter gene assay for their agoniztic effect on human nuclear receptors PPARα/γ/δ, RXRα, FXRα, RARα in HEK293T cells. Compound 1 was tested in an isothermal titration calorimetry (ITC) assay to measure direct binding affinity to the ligand-binding domain of PPARγ. We appreciate and respect other drug discovery tools, and our aim is to add a new perspective to drug design.
TransformerCPI2.0 still outperformed the baselines (Fig. 3c and Supplementary Table 6). This test is more challenging than the whole dataset because the drastic change in biological activity in this range involves the conversion of an active compound into an inactive one or vice versa. At last, we showed some illustrative examples (Fig. 3d) that subtle structural differences produce drastic changes in activity, when none of the protein targets and compounds were included in the training set. These results indicated that TransformerCPI2.0 can capture more useful information about compounds than baselines when training on the same dataset.
The detailed presentation of all of them is out of the scope of the current review but they are analysed and compared in several good reviews [40,41,42,52,53,54,55]. Synthetic Marijuana, also known as spice, K2, black mamba, and legal weed, is a designer drug consisting of plant material sprayed with synthetic psychoactive chemicals. Since 2009, K2 has been widely sold with a label warning that it’s not meant for human consumption. The challenge for rational drug design in our genomic era is to take advantage of knowledge generated by genomics and to convert it into useful therapeutic agents. The challenge is twofold because of the changes that are introduced by the massive information that is generated by both genomics and proteomics.
No comments:
Post a Comment